AgileGAN: Stylizing Portraits by Inversion-Consistent Transfer Learning
Guoxian Song, Linjie Luo, Jing Liu, Wan-Chun Ma, Chunpong Lai, Chuanxia Zheng, Tat-Jen Cham — ACM Transactions on Graphics (Siggraph 2021).
Fig. 1. Top row (small): some sampled style exemplars. Bottom two rows: input images, results from Toonify [Pinkney and Adler 2020], and our results for multiple styles. Given a single input image, our method can quickly (130 ms) and automatically generate high quality (1024×1024) portraits in various artistic styles. For a new style, our agile training strategy only requires ∼100 style exemplars and can be trained in 1 hour.
Abstract
Portraiture as an art form has evolved from realistic depiction into a plethora of creative styles. While substantial progress has been made in automated stylization, generating high quality stylistic portraits is still a challenge, and even the recent popular Toonify suffers from several artifacts when used on real input images. Such StyleGAN-based methods have focused on finding the best latent inversion mapping for reconstructing input images; however, our key insight is that this does not lead to good generalization to different portrait styles. Hence we propose AgileGAN, a framework that can generate high quality stylistic portraits via inversion-consistent transfer learning. We introduce a novel hierarchical variational autoencoder to ensure the inverse mapped distribution conforms to the original latent Gaussian distribution, while augmenting the original space to a multi-resolution latent space so as to better encode different levels of detail. To better capture attributedependent stylization of facial features, we also present an attribute-aware generator and adopt an early stopping strategy to avoid overfitting small training datasets. Our approach provides greater agility in creating high quality and high resolution (1024×1024) portrait stylization models, requiring only a limited number of style exemplars (∼100) and short training time (∼1 hour). We collected several style datasets for evaluation including 3D cartoons, comics, oil paintings and celebrities. We show that we can achieve superior portrait stylization quality to previous state-of-the-art methods, with comparisons done qualitatively, quantitatively and through a perceptual user study. We also demonstrate two applications of our method, image editing and motion retargeting.
Video
Demo
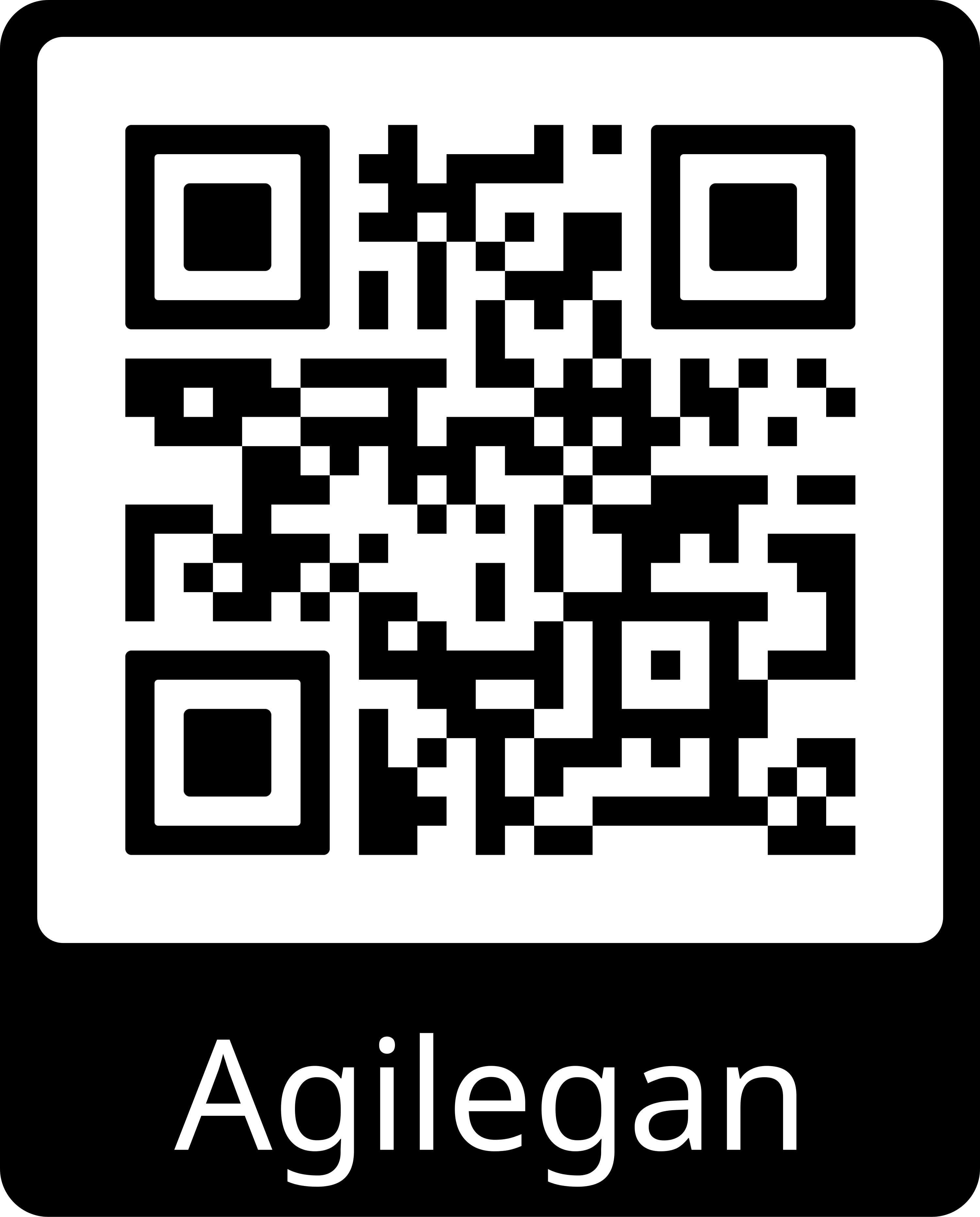
Please use phone to scan it, or you can directly visit the demo website.